Ep. 567 w/ Brian Stevens CEO at Neural Magic
Welcome to Building the Future, hosted by Kevin Horek. With millions of listeners a month, Building the Future has quickly become one of the fastest rising programs with a focus on interviewing startups, entrepreneurs, investors, CEOs, and more. The radio and TV show airs in 15 markets across the globe, including Silicon Valley. For full showtimes, past episodes, or to sponsor the show, please visit building the future show dot com.
Kevin Horek:Welcome back to the show. Today, we have Brian Stevens. He's the CEO at Neural Magic. Brian, welcome to the show.
Brian Stevens:Thanks, Kevin. I'm happy to be here.
Kevin Horek:Yeah. I'm really excited to have you on the show. I think what we're gonna talk about today is actually really innovative, very cool. Selfishly, I'm really excited because I'm doing some stuff in the space, and I really want your opinion on a bunch of things. But before we dive into all that, let's get to know you a little bit better and start off with where you grew up.
Brian Stevens:Yeah. I grew up in, in a small town in upstate
Kevin Horek:New York, close, Chicago. Okay. Very cool. So walk us through you went to university. What did you take in wine?
Brian Stevens:Yeah. Like, the, I'm probably gonna date myself, because, you know, like in high school, you know, it was really when, like, programming just started to become, you know, early parts of like high school curriculum. And so I was able to, I was able to, dabble is the right word, but, you know, get a little bit of experience, you know, on that front. But I but I thought it was, just a fun class to take, like Okay. Create or or create.
Brian Stevens:I didn't think it was could ever be a curriculum. And then, move from, we moved the family grew up, in New England. So even though we were kind of spending a lot of time in New York, you know, born there and went through high school there, they wanted to get back to, my parents' roots, Maine, New Hampshire. So we, we moved back my senior year. And, and, but I, I, you know, the UNH thing kind of came out, came about backwards because I actually wanted to be a creator, but I didn't know that you could do that with code.
Brian Stevens:So I was trying to do it with, Wood, believe it or not. Interesting. And, yeah. And so so I had, like, this guidance counselor that I just, like, have to pay down how much I owe him because he just, like, steered me in this other field, which was computer science, which was just getting rocking. And and the University of New Hampshire actually had a really great emerging computer science curriculum and in part because they were, Digital Equipment Corporation.
Brian Stevens:You know, that grew up in Massachusetts. And so they UNH was kind of a feeder school of all their technology. And so just kind of fell into fell into it there, to be honest. Like, I was thinking about it for years and just fell in love.
Kevin Horek:Very cool. So walk us through your career, maybe just some highlights along the way Because you've done a ton of stuff, and I really wanna kinda dive into neural magic and what you guys are doing there.
Brian Stevens:Yep. Yep. Yep. Probably had, like, 3 main sections with the first was, you know, like I said, it was digital. They did all their operating system development in Southern New Hampshire, and so I joined that team, you know, right out of college.
Brian Stevens:And that was great because, you know, you really only got access to technology if you work for a big company. Like, the Sure. The whole of open force wasn't prevalent back then. And so if you wanted to, like, explore, then you had to work for somebody that had that capability. And then but it was great for a for a young engineer because I just, you know, just brilliant people that I, was able to spend my day with and learn from.
Brian Stevens:And so that that set me up, but I was a I was an oddball computer science, developer, CS developer in that. I really cared about the outcomes that technology was driving and the user experience that your audience had around those technologies. So I I kind of fell into that direction, and that, you know, fast forwarding, it led me to the future for enterprise wasn't gonna be, you know, these proprietary companies that were selling big expensive servers and proprietary software stocks. It was gonna be more along the lines of, like, commodity intel. And so that was the pathway that led me to to Red Hat, and then, just first principles looked at, one, what's a business model on open source?
Brian Stevens:But then I think more importantly in that is, you know, what do enterprises really need, from techno from, you know, technology stacks. And I don't mean just the features. I mean, everything else around that. And we focused on we focused on that and not just speeds and feeds. And then, and and and part of that was, you know, I was lucky to see, you know, the, you know, the public cloud's born.
Brian Stevens:Amazon was our biggest customer at the time, so I saw that, you know, front row seat. Did that movie and then, you know, went and joined Google for 5 years when they were just getting started and and building cloud and, went there, moved out to California, and, again, like, refocus them, you know, away from just serving tech companies with with cloud capabilities and and, you know, pointed that at enterprise. I just felt like the opportunity for what enterprises, you know, need from as a service, like, was unfulfilled. Did that for 5 years. Dad was diagnosed Alzheimer's back in New Hampshire and
Kevin Horek:Oh, sorry to hear that.
Brian Stevens:Get back in yeah. Thank you. So so that led the the a really quick transition back. Still stayed working for Google, but, you know, being remote pre COVID, you are the odd person out. So did that for a couple years, and then and then, yeah, kinda wrapped that up and had intended on just doing, some boards and actually writing some code.
Brian Stevens:And that was kinda where I was at, up until, Color began.
Kevin Horek:Okay. So walk us through, coming to Neural Magic and what exactly is it?
Brian Stevens:Yeah. So Neural Magic, was born out of MIT, and, the the 2 founders. And one of the founders, Nir Shavat, MIT professor, he focused, he what he teaches on, what he's expert at is is is really, he'll kill me the way I say it, but, like, really under really, you know, systems level, infrastructure and performance. So really understanding, you know, how computer systems and specialized processors work, and then how you build, that marriage between hardware and, software efficiency. And so, you know, really extraordinary person.
Brian Stevens:And then and then along comes, you know, the emerging field of AI and behavioral. And he started, you know, looking at how would AI meet computer systems. And the ideas he had was, was that you could that existing commodity CPUs, you know, Intel class CPUs, the things you, you know, running in your MacBook, could become, like, really great, processors for running, machine learning models. And that was really contrarian, back then. But that was really, you know, his and and his other co founder Alex's early ideas on, like, building a tech stack that made AI work really amazingly well just on an ordinary CPU.
Kevin Horek:Okay. So walk us through how you came to be there and and become CEO.
Brian Stevens:Sure. So my my plan was, don't ever take a full time job again and just get to explore and spend your time on the, you know, piece of it that yeah. Like and so I'd reached that point, and I was very happy. And then, unfortunately, I met, Nir, and and I just fell in love with him. I fell in love with, and I met him through a VC, you know, a Okay.
Brian Stevens:A VC friend, you know, of mine and, just had dinner with him, but with no intended you know, no intentions. But the but he's just, he's the kind of person that that you would love to spend time on with in any capacity, to be honest. That's awesome. And then, you know, I was always, like, the areas I spent my time on are areas that, like, that really trying to understand something that that I don't understand. Right?
Brian Stevens:I mean, it sounds obvious, but, like, but that really intrigues me. And what I realized in the space of AI machine learning specifically, it's everything you've learned made you realize there's so much more you have to learn. So you didn't feel like you're an expert, but you had I think the journey is you're never expert. And that's that to me was, you know, at this point, my career was really highly interesting. And, you know, it and with Nir taking that focus around, a contourium view around, you know, running on CPUs was how was really interesting.
Brian Stevens:And so it was, you know, the, committed a day a week, to help Neural Magic as they build a business. Did that for about a year year and a half. COVID hits. All of a sudden, I realized even though I only wanna spend a day a week with them, I've realized I'm spending 7 days a week. That's awesome.
Brian Stevens:And then, yeah. And he's a very persuasive guy and I was very passionate about the space. So, you know, after a year and a half of advising them, I I joined full time just about 3 years ago as CEO.
Kevin Horek:Very cool. So what exactly does neural magic do? And then can you maybe explain the concepts behind it without getting too technical? And because AI is everywhere, people hear that, and machine learning, do you maybe want to give us kind of, like, a high level of what you guys do, how it ties to that, and kind of where we're at in the space? Because I think there's a ton of myth around what's happening right now too.
Brian Stevens:Sure. I would say the if I kind of put the what AI can do aside for a second Sure. That's perfect. And, and I go and look and what I love now is, like, everybody has a really well I'd say pretty well calibrated on the capabilities of AI because, you know, even people that aren't in our fields, right, is experience experiencing it through, like, CAPT and other models. Sure.
Brian Stevens:Right? And so so that's really interesting. So I think people can see, these capabilities that didn't exist before. What we're trying to do at the highest level, like, from a mission perspective is bring those capabilities to, enterprises, but bringing the capability in. So in many ways, like, that way to explain neural magic is to first talk about just the capabilities of AI that have really exploded, you know, in the last, well since November of of 2022 when OpenAI made the Chachapiki model.
Brian Stevens:So it's really easy for people to see these capabilities around especially around large language models that didn't exist before. And what we're aiming, there was major breakthroughs on that. So, like, on on how they got there, which is really, really compelling. But what Neuromagic is aiming to do is to enable enterprises to use that capability, but to do it in a way where they completely control their own destiny. And so it's really back to, you know, the open source roots, you know, that I, you know, grew up on with with Red Hat around the the the control and flexibility that open source brings to, end users and enterprises.
Brian Stevens:And so one would argue, like, well, yeah, but there's no open source capabilities in the in the AI space. Those are only, like, the big tech companies. That's actually not true. So, like, the an amazing, group of of AI models for large languages, has been developed and, in open source with really permissive licensing. And the innovation rate of these new models, you know, they get they get more accurate, they get faster on a month over month basis.
Brian Stevens:So there's there's great, set of choices that people have to own their own AI model. And what we're aiming to do is to help them use those open AI models and, be able to optimize them in such a way that they can run anywhere that an enterprise would want them to, whether that's, you know, in their in their cloud zone or whether that's inside of their an existing data center, whether it's to run inside of a brick and mortar, location. You know, once the model state of the art is opened up, it really puts, you know, customers and enterprises in control of their destiny. And the value of that is, you know, they get pure privacy. They can 1, they own the model.
Brian Stevens:2, they can customize the model as it makes sense, on datasets that make sense to their use case. And then they control, like, the terms of deployment locations and their choice of infrastructure. So so it can be this really liberating future where where you'll get the best capability, but you'll get it on your terms. And where neural magic comes out of that is, there's these models are massive. Now Sure.
Brian Stevens:Okay. L and large language models. So and that was the breakthrough. The prior world was, you know, large language models wouldn't actually behave any better than smaller models from a from a capability perspective. And that was actually turned out to not be true.
Brian Stevens:So they're amazingly capable, but they're really hard to run just because they're so big. And so that's why the world, let's say, without neural metric has been heading down this pathway that you gotta, like, buy really expensive infrastructure to run the models on. And that infrastructure is prevents a lot of optionality, you know, that customers would have otherwise. And so we get in and we optimize the models, and we build deployment capability that lets the models run, like, super efficiently across, all infrastructure choices. And then and then so you can you get to pick, you know, where and on what you wanna run your AI models on.
Brian Stevens:And that's really important, because if you really believe this world where these AI models are gonna be parts of every application, and that's kind of what I subscribe to in the future, they're just gonna be libraries and then you're gonna use AI capability, you know, across the stack. Then, you know, you need the flexibility. You want that to feel like just any other application and not a piece of the app that has to have, like, really expensive serving infrastructure in order to run it.
Kevin Horek:No. I agree with you. I I think it's gonna be in everything, and it just I think it makes a lot of sense for a lot of things. But but I'm curious. Okay.
Kevin Horek:So if I'm a large retailer or a bank or or, like, a enterprise company, how do I actually start using neural magic? Like, do you guys consult and kinda come in and look? Or, like, how do you figure out where I can actually implement the technology and where I should be using AI? Sure.
Brian Stevens:And so the the where I should be using AI, most enterprises are already down that road.
Kevin Horek:Okay. Oh, interesting.
Brian Stevens:So they're already down the path of, what you just said is use case selection. So where are, in some cases, the high valued ways I wanna integrate? Typically, it's vision or language models.
Kevin Horek:Okay.
Brian Stevens:My existing enterprise. And so, and so they're already so we we really meet them where they are, where but I'll be at with, you know, the vision ones are you know, have been going on for years. The large language models are really have been only going on for, like, the last 6 to 9 months. But so we work with them once they've, defined the set of use cases that they want to use AI for. And then we work with them to, you know, really shoulder to shoulder to how to optimize their model simply, in a way that gives them the flexibility to run it on, you know, their preferred piece of infrastructure, you know.
Brian Stevens:And so and then often part of that too will come help with in most cases, they wanna fine tune their AI models because, like, if you, like, a good example is look at the, OpenAI models. They're really amazing generally, but they they won't have a level of specificity that might be important to an an end user. And so to get that level of specificity, like one example that uses, like it might know what a cucumber is, but it might not know the, you know, the the condition, shape, size, etcetera, like, of a cucumber. And Right. So what enterprises do if you're in the cucumber business is they can actually fine tune and train their model, you know, that existing model, like, to know that level of specificity.
Brian Stevens:Did that make sense for their use case? So we can help them with that fine tuning. But most importantly, we take these large language models, and we we shrink them down significantly that in a way that keeps their accuracy. But the the shrinking of the model means, as we were talking about before, these heavyweight models are hard to run, all of a sudden become much smaller models that are easier to run. So that's a big part of kind of magic that is neural magic is how do you actually make these models smaller but not lose any of the capability.
Kevin Horek:Right. Okay. And then how do you work with them kind of on a hardware optimization space? Because we all know that is part of the big challenge with AI right now too.
Brian Stevens:Yep. And it and it's the 2 parts. So the the first part is apply a set of model optimization techniques to their existing model.
Kevin Horek:Okay.
Brian Stevens:And that makes the model smaller. And it it's obviously way more complicated than that. But, like, but it's it's not from an and the bridge user where, like, how that works is kinda rocket science. And then and then the second part is then what we are able to do is we're able to help them size. So the, really, it means, like, choosing the right infrastructure, in this case, it's CPUs.
Brian Stevens:Do you wanna run on Intel, AMD, ARM? What size of processors do you need? How much memory do you need? Aspects like that. What generational hardware do you can you do you wanna use something that is legacy hardware that you've, you know, already have in your data center?
Brian Stevens:Or do you want to, like, take advantage of some of the newer capabilities of CPUs that are coming out that have, like, AI friendly instruction sets coming into them. So there's definitely, help them with sizing and then help them with optimization, at runtime. Because we also have a software stack that runs on the CPU, that runs these models in a really performant way. And so there is a there is a a path where we work with them generally to do further optimizations at deployment size at at deployment time.
Kevin Horek:Okay. So how does it work then from your side? Like, do you just bring in, like, different members of the team based on what the user is trying to do? Do I just basically go to your GitHub and and start implementing? Or, like, walk us through
Brian Stevens:that. Yep. So, like, the the it's definitely a a product led, experience. Meaning, what we didn't want is we didn't wanna have to have the call sales button on the website.
Kevin Horek:Right.
Brian Stevens:Yeah. Right? Like and so what and which is fine. It's a great choice for many, but, like, we wanted to build a open community that, enterprise developers or otherwise, can just join our Slack, right, and actually get started. And so we try to make the product capabilities, the documentation, the how to's all available.
Brian Stevens:And then and then as as people join the Slack, then and they have they can just go there for help on how to get started. They can go there, when they have challenges. And so we meet them in Slack first, the community. And so there's thousands of, you know, machine learning engineers that live in the Slack community and we help them there. And then there's also enterprise pathways because, you know, sometimes that's not necessarily the best, pathway for for certain enterprises.
Brian Stevens:So then the enterprise pathways are such that, you know, we really look like a extension of their machine learning engineering team. So it's definitely the capability we have are codified through, software products, You know, when for the one, you know, set of software tools for the optimization piece, and then one set of software tools for how, you know, running it on CPUs in production. But still though with that, like, the best thing you can do is to, you know, help, you know, machine learning engineer engineers that are getting started or this is new to just help them shoulder to shoulder and act like an extension of their r and d team. And that and that's what we
Kevin Horek:do. Got it. Okay. You have some demos and examples on your GitHub page. Can you maybe give us some examples of how people have leveraged the technology just so, you know, people can say, like, hey.
Kevin Horek:I could actually use that.
Brian Stevens:Yep. The vision is definitely further along, you know, in terms of because it's a more mature space. Sure. And so like the you know, for years, people have been using vision and neural magic for vision, use cases. And then the large language models are really just, you know, that's where all the fervor is right now.
Brian Stevens:Right? Okay. This is because it's going to have a larger impact on types of things that large language models can do for enterprises from operational efficiency, etcetera, etcetera. So but they're definitely earlier on that journey, with or without Neuromagic. But on the vision side, like, oh my gosh, customers, like, doing, you know, self driving car lane detect well, not necessarily self driving car, but lane detection.
Brian Stevens:So pretty sure your vehicle's gonna have lane detection. Right? So imagine sticking, like, a big heavyweight power hungry GPU into a car. Not just for the cost, but just the power consumption and the failure rates, you know, etcetera, these things have. They're not designed for that.
Brian Stevens:So the ability just to run your AI model on your existing CPUs, you know, that might that are already in cars for doing lane detection is, like, one really powerful use case. Interesting. It also includes, you know, airplane use cases that are in there around for vision. Another set of eyes is always a really great thing as we know. Sure.
Brian Stevens:When you're taxing or otherwise. Retail stores, a lot of, using, like, RSTACK and just generally retail stores that, are are, in some cases, helping with shrinkage. You know, it just, you know, positive as if we're gonna give you a better experience as you do your own self checkout, but also, you know, helping, you know, I'm not sure that's a better user experience you have, but it's definitely deployed to help reduce the amount of, you know, shrinkage or theft, right, that retails have. So that's happening generally across retail, including at, like, the, you know, as you walk out the door. So number a number of things like that, just anywhere you can imagine, like machine intelligence for vision.
Brian Stevens:There are usually cases at the edge that GPUs are are are difficult.
Kevin Horek:Okay. And that that's that's really interesting. I'm also curious to what are your thoughts on kind of the large action model stuff that's kind of coming out too? Are are you guys gonna ever go into that space or or what are your thoughts on that space?
Brian Stevens:We we haven't yet. Like, everything's really been large language right now. So we Okay. We had the the last year, and we're just, about to bring out support for that. And the reason being is the way the way large language models process, they're even more taxing on infrastructure.
Brian Stevens:So as much as, you know, so the state of the art, for for natural language processing, there were smaller models before. And they didn't have the capabilities that these new large language models have, like these open source LAMA, open source mies trial. These are really exciting new state of the art models that have the capability of what you'd see come out of, you know, the big tech for serving APIs. But they process completely different. They're really, they actually need more infrastructure, to run these, because not only the amount of compute, but the, they really press on the memory, requirements and memory bandwidth requirements that, you know, that that you need to run these.
Brian Stevens:And so, like, a lot of our tech stack, was rebuilt to support large language models specifically, so that you don't need to so you can run them on CPUs, so that you can run them on even, you know, GPUs that have less memory or smaller GPUs. In many cases, you know, you can use our optimization techniques and you won't need a big GPU. You can use a small GPU. So all that's really gives enterprises optionality and it's and it's really liberating. It lets them use AI in use cases that, that are more pervasive because the cost is reduced.
Brian Stevens:No.
Kevin Horek:That that's really cool. So I'm curious to get your thoughts on you you mentioned something earlier about, like, a lot of companies that you guys have been working with actually have what they wanna do with AI. I've seen, like, where yes. That's true for some companies, but I've seen where companies maybe wanna implement it, but they don't really know where to start or how to go about doing it. What are your thoughts around that, and how can neural magic, like, help them actually maybe go through that discovery a little bit more?
Kevin Horek:Because let's be honest, not all of us are technical. And I think not a lot of people have still kind of played with AI or maybe they have and they don't know they have. Right?
Brian Stevens:Yeah. Yeah. No. That's true. And then, like so we, because we are, even though we focus on the optimization aspects of that, So existing models, that that we optimize and make them run anywhere.
Brian Stevens:The reality is we have the experience of, I'd call it like the the art of the possible.
Kevin Horek:Sure.
Brian Stevens:So so if you looked at large language models, what what are a reasonable set of expectations, that that a end user could have around the capabilities of these new AI models that are coming out? And so we can very much help them with that. So Okay. Obviously, the the part that they need to do is to really understand the, the, you know, the grounds up set of use cases they wanna consider. And then we can help them down select that into some Okay.
Brian Stevens:Choices based on the AI capabilities. And, you know, one of the, public companies that I'm on the board of did something similar, you know, where they they across their space and the way they work with customers, they they looked at over a 1000 use cases. You know? So they Oh, wow. Outsourced a 1000 use cases of the types of things that AI could do, and then they down selected that into the 10 based on the capabilities of large language models today.
Brian Stevens:And that I think is a really very reasonable choice because you said it best. I'd say, like, this is it's a world where everybody's doing it, but not everybody's, like, delivered to production yet. Yeah. Right? Because I think it's, like, you know, there's skills that are needed as well, right, around this and and assembling your machine learning engineering team, you know, that has ability to, not just assess the use cases, but then also get the right model into production and maintain it.
Kevin Horek:No. That that makes a lot of sense. So I really wanna talk about, maybe this is kind of high level, but it seems like if you read the news, it's like, you know, AI is taking over the world and gonna destroy it, which is no worse. Like, I don't think that's ever gonna happen, but we are so far from that ever being an issue that, like so, like, where we actually at with, you know, kind of AI and machine learning? Because I I think there's so many miss out there and, like, there's so much paranoia around it.
Kevin Horek:So can you maybe, like, talk through that a little bit?
Brian Stevens:Yeah. I think, like, the, I think a lot's changed in in, you know, a year. Sure. We weren't talking about these things a year ago. So the the the capabilities I worry less about, you know, the setting an AI model, right, that's learning and smarter than people.
Brian Stevens:Yeah. Part that keeps me up at night is because, just like these, even the open source AI models can help enterprises, become more efficient. Right? What used to take a knowledge worker to analyze data, is like now all of a sudden the knowledge worker is just making the decision the data is analyzed for them, like, in a really meaningful way across, like, large volumes of data. Like, those, like, knowledge workers have just been, like, completely empowered A 100%.
Brian Stevens:Through the use of of AI. And that's amazing because it's all around, like, you know, you know, having more output per person. Right? So I don't look at this as the world where you don't need people. I look at the world where the, the business output per person is just gonna go up much higher.
Brian Stevens:And that's an amazing thing. But I do worry I do worry less on the sending aspect and more around the fact, like, you know, if if we've, you know, also armed, you know, the bad people. Right? So, like, in my eyes, just because I think, like, you know, like, if you're trained in technologies, you can look at an email, And you can look at it and know that, okay, that's a phishing email. That's, you know, not there's no way.
Brian Stevens:Look at the URL. There's just different aspects of it, right, that you can look at and know, like, it's spam, it's fake, and, you know, don't click that link. That's gonna become next to impossible. Yeah. Down the road.
Brian Stevens:And that's I think it's area so you need so it's gonna be a security arms race as well because the quality of the bad people are just gonna get they're gonna get a, more robust set of tools. Right? It'll actually really look like you know, today, like, the faking PayPal stuff looks like fake PayPal. Yeah. Yeah.
Brian Stevens:100%. This world down the road where, man, that looks really incredible. And so that's the stuff that I think worries me the most in the next, you know, number of years.
Kevin Horek:Yeah. That's that's an interesting point. But to your point before that about, like, it's changed my workflow immensely and just saved me a ton of time. Like, I moved my default search engine away from Google just to one of these, like, chatbots now because it's quicker and it gives me better things. And then I'm generating design ideas with, you know, AI sometimes.
Kevin Horek:And, sure, it maybe only gets you 20 to 80% of the way to a screen. But if I can just you know, on the days you're not feeling creative, if it can just spark some creativity quickly and you can iterate from there, like, and then you can summarize things and make me a better writer. Like, it's it's not really taking away. It's taking away maybe some of the boring parts or the parts I hate or the parts I'm not good at, but it's not wrecking my job, at least not today.
Brian Stevens:Yeah. Like, you're more you're more effective. Right? I mean, maybe it's better quality, but you're certainly more effective, you know, and your output, you know, that you get done, You know, it's amazing, like, even in your design background. Yep.
Brian Stevens:Like, you starting from, you know, some you know, you can you can probably have like a software tool that you now can, you know, develop you the some of the starting designs that you want. Not so it's not just it's not just, you know, text. And, and I think that's really powerful. And so, so I agree. So like, like you've, you're further along than I am.
Brian Stevens:I've, I've used all of the the models. It hasn't changed what I've done in search. It's just become a, a daily tool that I used, for different tasks, together with search, at least the types of things that I use search for. So it's just like yeah. So now it's not just one thing goes to the next.
Brian Stevens:It's just like we've just we've been armed with this amazing tool that's gonna make us, much more much more productive.
Kevin Horek:Well yeah. And, like, even just something as simple as, okay. Like, I I have a design, and then I can test it through AI, like, a heat map thing. And then out of Figma, you can get it to write, you know, react or whatever code you want. Sure.
Kevin Horek:It's not a 100%, but it's better to give the developer 80%. Maybe they're gonna have to rewrite some stuff than them starting from scratch. Right? And then I can go in and tweak like, it's changed my workflow immensely. Right?
Kevin Horek:And I think if it can it it's obviously only gonna get better. And if people I think the big thing is, and I want your opinion on this, is it's coming whether people want it or not. It's already here arguably. You basically need to adopt these tools to make yourself better than trying to say, like, no. Don't do that because it's gonna wipe me out.
Kevin Horek:Right? You're gonna have to just embrace it.
Brian Stevens:Yeah. And I think I think that's true. Like, you were saying, like, if you had to back up the clock, what degree would you pick? Right? Yes.
Brian Stevens:Is your is your is your, the thing you pick going to be automated away or is it going to be empowered? And I'm multi camp, like all these degrees ago, I'm just, maybe I'm contrarian, but I'm on the camp like these, these, the, the jobs are still going to exist, but man, you're going to be just supercharged, you know, not just, like out there with a, you know, back at, back at your HOMV, the comp, just the you'd write a 1,000 line program. Right. And back then, like just the taxing that you had on the infrastructure, it could take it an hour to compile that program. So the reality of it was, and so look at today's computers, like you can file in seconds.
Brian Stevens:So today people use the, you know, they don't have to write quality code because the compiler like catches everything right away. We had to like really the tax of having a bug in your code was really high Yep. Because it caught for an hour a turn. So we got we got really good at, like, developing software first, you know, developing a program first out of the gate. So I look at it as, like, that you just said, like, the the design tools, the writer authoring tools, the the code the the open source code models, and there's, you know, the the paper token ones like GitHubs and stuff like that.
Brian Stevens:But the the the open source coding tools are really popular. And and if all of a sudden you can accelerate and generate half the code, you know what I mean, that a developer would have, you know, that a developer uses. That's really powerful. It's only gonna get better. But, yeah, they're still gonna be there as a job.
Brian Stevens:They're just gonna, like, get way more done, and you're gonna end up being you're gonna have the company that you work for or the start up you work for is gonna have a even more robust set of tools, but you'll still exist.
Kevin Horek:Sure. A 100%. Well, even some of the no code code tools now or, like, the barrier to entry into the space now to even build your own startup. Like, Flutterflow is really good, and, you know, Bubble's not bad for certain things. Like, there's a bunch of really good no code tools.
Kevin Horek:And then the crazy thing about it and I'm not a developer, like, we kinda cover throughout the show. Like, I built my own chatbot in Flutterflow connecting to chat g p t. Like, sure, it was a tutorial online, but 2 years ago, I would like, it would not have been possible for me to do that. Like or I would have struggled so hard and needed, like, so much time, and what I built in an afternoon just wasn't possible for me to do, like, 2 years ago. And why I'm bringing this back is because I think what you guys are doing at Neural Magic like, anybody can leverage the technology that you're building, whether you're technical or not, because I think there's so many tools that you could just leverage to to use, you know, your basically large language model back end, basically.
Brian Stevens:Yeah. Yeah. Yeah. Like, I mean, there's there's there's it's gotten so much easier, and we're only a few years in. I I love the I mean, the in the low code, like, that's a great example because, like, the the low code was trying to solve the same problem, make development more efficient.
Brian Stevens:Right? You just shouldn't have to be, like, understand how to build a full stack program. Right? Like, it just doesn't make sense. And so the mission of of of, you know, AI helping that process, you know, they're they're really kindred kindred spirits and, like, yeah, like, I I just don't wanna see this world where AI is used for the most highly valued use cases because they're it's really expensive.
Brian Stevens:And so it has to have an ROI. So I don't want and you just have to do an ROI analysis of, can I afford, you know, whether it's the infrastructure or paying per token? Right? Like, I want to eliminate that and just let AI be used everywhere that's helpful. And you're not making a cost analysis of whether it delivers the value.
Brian Stevens:You know what I mean? For the cost that, you know, it takes to, to deploy it. And I just think like, and so again, it's much it's very aligned with open source and, you know, let's just go commoditize this whole space around the these deep models, you know, not just the frameworks Yeah. But deep models and make them easier for people to use and adapt.
Kevin Horek:That's that's actually really fascinating. So how does Neural Magic monetize then?
Brian Stevens:We, so we so everything around the, ways you optimize models
Kevin Horek:Okay.
Brian Stevens:We just pay open source. So, like, so pre deployment. So there's a lot of techniques around, quantization that are out there that we've led the, all the research on these new, you know, the the best quantization arms are out there as well as, what we're known for is also sparsity, which is how do you, like, 0 out a lot of what's called the model weights that these things have. And if you can zero out 80% of the model weights and keep, you know, 99% of the accuracy, then you got something. But that side, we've all open sourced that.
Brian Stevens:So all these tools and research techniques on how to optimize a model we've open sourced. And then, what we monetize is when somebody, gets to the point where they wanna, put these AI models to work in production use cases.
Kevin Horek:Right.
Brian Stevens:They will monetize the deployment side engine,
Kevin Horek:Okay. That
Brian Stevens:runs on the CPUs, you know, so it comes by way of a subscription with support and, you know, you know, everything around, helping people with the, you know, their deployment stack and the models they're using, etcetera, etcetera.
Kevin Horek:Got you. That that makes sense. And it's just like a paper usage kind of thing, or how does that work?
Brian Stevens:It's a paper package, so it can be sized based on the type of problem you're solving. So the easiest way because it accelerates, model optimization, so, you know, I mean, it can make a a set of CPUs 4 to 12 x faster is you just take a tiny piece of that, you know, the efficiency that you could even back through way of subscription. But then the value you bring to them is, develop a partner, you know, and a support partner, that helps them on their journey.
Kevin Horek:No. Makes makes a lot of sense. So you've been in this tech space a long time, some very big roles at some very big companies. What advice do you give to people that are maybe just starting out, people that are been in the industry a long time, and and just any other advice that you've kinda learned along the way that you'd like to pass on?
Brian Stevens:Yeah. I think I think what, you know, what helped me a lot was, especially on the engineering side, is don't get buried into the technology. Really, really understand, you know, the experience that you're creating, you know, for an end user as consumer enterprise, etcetera. Right? Doesn't matter.
Brian Stevens:But just really understand the problem you're solving, the experience that your end user would have in using the technology and focus and be manic about that. It doesn't matter what you build. And I think it's I think as as software developers, it's often too easy to get caught up into the the software part itself. That's not an ultimate problem that you're trying to solve for. And so that ends up what it means is you end up wanting to become super user centric.
Brian Stevens:Right? Right. Conversation, you know, that's what I always love, like, all the user research. Like, you wanna be the best way is to be, I think, be a developer, but then innovate and increment vastly, quickly, you know, through a direct relationship with your users and advance that along a lines that, make sense for them.
Kevin Horek:No. I I think that's actually really good advice. And I think the nice thing about what you just said is, like, whether you love Apple or hate Apple, they basically made that their business model. Right? Like, they it's not perfect, but, like, they were the one of the first companies to say, like, we really care about user experience.
Kevin Horek:And that doesn't necessarily always mean just, like, the interface. It's like from from everything they do, it's all about the user and the customer and trying to make them happy. It's not perfect, but, like, I think that makes a lot of sense. Because in my experience, and you could tell me if you're I'm wrong here, is it doesn't matter if you have a 1,000 features. If nobody gets past the sign up box, it doesn't matter.
Brian Stevens:Yeah. Yeah. And people in it that's that's why I love like, I've always said, like, the user experience starts with discovery. Yeah. Right.
Brian Stevens:It's not just like once I installed it and how it works. And like you said, people often like think, and it must, it must kill you. Like the user experience is just Yep. So Milo was like, how did they discover us? Like, you know, what did they first see?
Brian Stevens:How did they try? How did they procure? Like, what was the what was the contracting? I mean, big part of work to Google is making contracting so simple. Like, what is the experience?
Brian Stevens:Like, the whole user journey, you know, like really matters. And so I even started, like, you've mentioned apple, like when I buy a consumer product and I open it up, I like spend my time on the packaging and like, oh, he is to open it. Did they get that part right? Like, I just think it's the whole end to end experience, and I think too few people, don't think that way, unfortunately.
Kevin Horek:Yeah. I I always tell people that I think everybody at a company should be doing user experience because Yeah. If if you don't have customers, everybody goes home or has to get a new job. Like, that's the reality.
Brian Stevens:Yeah. We're all I always said we all work for sales. You know? Yeah. And then and that was like like, why, like, you've talked about, like, Apple, like, their early d school stuff at Stanford was some of the things that I tried to emulate, you know, back at Red Hat Interesting.
Brian Stevens:Long time ago. You know, just like getting back in touch. You know what I mean? With with who your users are and and and meeting their needs.
Kevin Horek:Sure. But, Brian, we're kinda coming to the end of the show. So how about we close with mentioning where people can get more information about yourself, neural magic, and any other links you wanna mention?
Brian Stevens:Yeah. Like like, I know we went through a lot. Like, I think the the the the beginning user voyage is is to come to, you know, neural magic.com. And, and there you can jump off. Like, you can jump off into the community if you wanna go the direction or you can just, you know, set up a set up a session and and, you know, one of our engineers or sales team will kinda take you through what we do and how we can help.
Brian Stevens:And so we're we're very much around meeting people, where they wanna be right now and where they wanna be in their journey, and then helping them with that.
Kevin Horek:Perfect, Brian. Well, I really appreciate you taking the time and your day to be on the show, and I look forward to keeping in touch with you and have a good rest of your day.
Brian Stevens:Thanks, Kevin. Enjoy that.
Kevin Horek:Thank you. K. Bye.
Intro / Outro:Thanks for listening. Please visit our website at building the future show.com to join the free community. Sign up for our newsletter or to sponsor the show. The music is done by Electric Mantra. You can check him out at electricmantra.com and keep building the future.
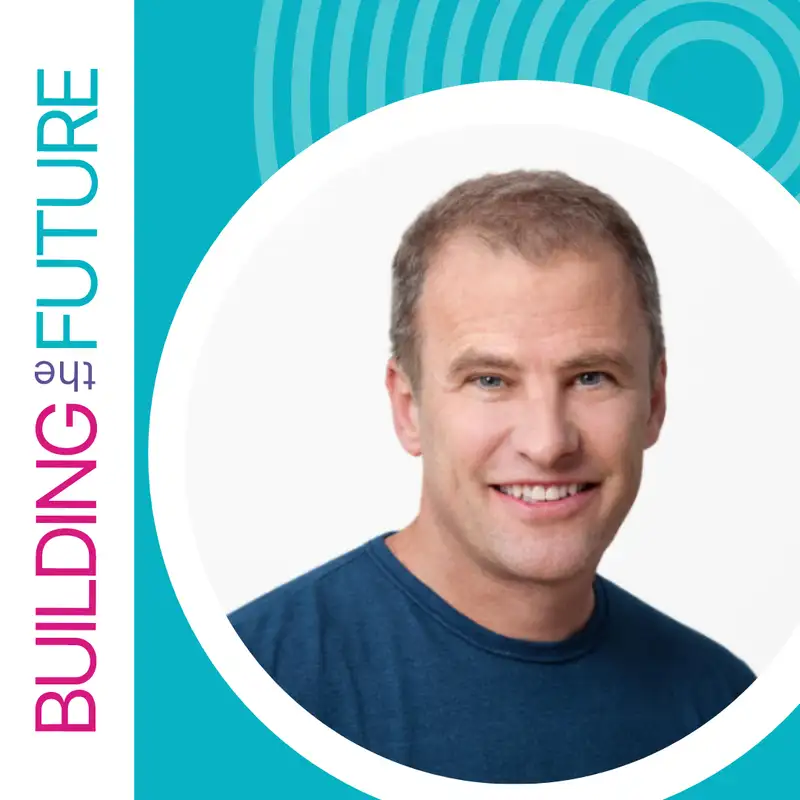