Ep. 539 w/ Sridhar Ramaswamy Co-founder at Neeva
Kevin Horek: Welcome back to the show.
Today we have Shaar Ramaswami.
He's the co-founder and c e O of Neva, and
he's also a venture partner at Greylock.
Shaar, welcome back to the
Sridhar Ramaswamy: show.
Super excited to be back.
Kevin.
Thanks for having me.
So much has changed in the last year.
Hard to believe.
Totally,
Kevin Horek: and I always love
chatting with you because you have
such an interesting background and you
actually were nice enough to let me.
Beta tests Neva very early on, and
I've been using it for, I don't
know, a year, year and a half or so.
That's right.
Every day.
I will say, and I've been using the
mobile apps as well, but maybe before
we dive into Neva and all the new
stuff you guys have been working
on over the last year, do you wanna
maybe give us a quick background on
yourself just for people that maybe
Sridhar Ramaswamy: don't.
Totally.
Uh, I am a computer scientist.
Uh, I am a proud immigrant
to the United States.
Came to get, uh, my doctorate,
uh, a long time ago, uh, academic,
uh, turn software engineer.
I worked at, uh, research labs, uh,
places like the famed Bell Labs.
Where the transistor was invented
before moving out to the valley about
20 years ago, uh, early employee of
Google grew with, uh, the team started
as an engineer actually before.
Uh, moving to more of a management role.
Um, incredible journey there.
Uh, helped grow the ads
and commerce, uh, teams.
Uh, grew my team from like, basically
myself to running a team of over 10,000
people making over a hundred billion.
Uh, and, uh, uh, started niva about four
years ago because I wanted to, still
wanted to work on search, um, but wanted
to do it very, very, um, differently.
Yeah, so I'm.
Um, you know, computer scientist, uh,
immigrant software engineer, proud dad.
That's awesome.
Kevin Horek: Very cool.
So, how did you come up with the idea
for Neva, and let's cover how it's
kind of evolved to what it is today?
Because the AI stuff that you launched
earlier this year is awesome, I must say.
Sridhar Ramaswamy: Thank you.
Uh, so we started Neva four years ago.
Um, we started it because we thought
that, uh, uh, ads driven commercial
search, so to say, uh, had sort of
reached the limit of its innovation.
Little did we know, uh, how true
that would become, but we said, aha.
If we started with no constraints,
I bet we can do better.
And so we created Niva as a user first.
Ads free private search engine.
We said we are going to monetize
only with customer subs.
And, uh, building a search
engine is really hard.
That's what we did in the first
three years of our journey.
Uh, early last year, we realized
all of a sudden that, uh, AI
would be a big differentiator.
Um, and, uh, it's really let us
propel, um, the product forward.
Uh, Neva Edits core is search that puts
you on the user and only you at the
center, and goes about creating a great.
Very
Kevin Horek: cool.
So walk us through, I get, it's a
little bit hard audio only to talk
about something visual, but how have
you integrated AI into nevas search
Sridhar Ramaswamy: results?
Yeah, so, uh, I am sure all
of your listeners, uh, by now
have heard about Chad g p t.
Perhaps they have even played with.
Uh, if you have not, uh, played
around with Neva or chat g p d,
you should definitely, uh, do that.
Uh, basically these are very, uh,
you know, chat g PT is based on
what's called the language model.
It's a machine learning model that's
been literally trained on like every
document that, uh, OpenAI could get.
It's, um, uh, it is,
uh, incredibly prolific.
You can ask it any question,
it'll give you answers.
But here's the catch.
Um, these language models
do not understand, um, you
know, what is trustworthy.
They think all documents in the
world look the same to them,
whether it is written by a domain
authority or written by a huckster.
Um, and so they sort of
get a lot of things right.
Instead of typing inquiries and
getting links, you can just ask
a question and you'll be shocked
at how good some of the answers.
Um, but because, uh, you know,
Chad, g p d does not have.
An underlying model for what is right
and wrong, what is recent, et cetera.
Um, it is not a very reliable
source of information.
Uh, you know, as computer scientist, uh,
euphemistically call it hallucinating,
it basically means like it can make
up things that are simply not true.
Um, it's an excellent writer, by the
way, so even if it makes up something
that is not true, The language that comes
out is very, like, believable language.
There are no grammar
mistakes or things like that.
Um, and so we looked at this technology
and we realized that it had incredible
power to understand what we as humans mean
when we type a query or ask a question.
Um, but just as importantly, these
models have incredible power in
going through webpages, in going
through content and figuring.
What are the right portions that
are relevant to a question, to
a query, and then being able
to summarize huge quantities of
content into something succinct.
So the goal that we set for ourselves
with Neva is we said we want the
fluency, um, the flexible nature of
large language models to be integrated
with search, but we also want search
that is authentic, that is believable.
That is referenceable.
We don't wanna say something
and, and basically tell you,
you should take our word for it.
We want to point, uh, you to the site
where we got that information from
so you can go there and look and make
sure that what we are saying is right.
It's a self-correcting mechanism.
So that's what we set out to do.
Uh, it's been two, three quarters,
uh, and what we released early this
year, um, was the first example.
Of a search platform built from
the ground up that is able to
do referenceable, real-time ai.
This means what does it mean in practice?
In practice, like with
all other great products?
It's magical.
You are, you put in a question, you get a
short 8,000, um, 150 word answer that gets
to the heart of what you are asking for.
Gives you a fluid answer,
but also gives you citations.
So if you want to learn more,
you can click on those, uh, and
uh, and, and go to those sites.
We are continuously working on, uh,
increasing the coverage of this ai.
Um, but the end product is simple.
Um, it has fundamentally changed the
paradigm of search from giving you a
bunch of links to giving you an answer.
In fact, that's our vision.
Search is going to be answers, not links.
Kevin Horek: Makes, makes total sense.
And the, the use case that I always tell
people that I find really useful, um, is
just take for example, Steve Jobs, right?
Obviously, like if I just want to know
something quickly about him, I'd usually
have to go to like Wikipedia or whatnot.
Now I just like type him in
Neva, the top of the thing.
It gives me like a quick overview
of who he is and, and kind
almost like instead of going.
A third party site.
I can just do a lot of stuff inside
Neva I, I guess, and I love that, right?
That I like.
That's right.
You're saving clicks and time for
people, but how does that affect.
Traffic to the sites that
you're pulling content from?
Because like obviously if some, some
of them probably don't care, some
care because they monetize with ads
and kind of everywhere in between.
So how do you kind of
manage that or handle that?
Sridhar Ramaswamy: Well, first of all,
um, I'll point out that with J G P
T, there are no citations whatsoever.
All traffic starts and ends with chat.
J p t.
Um, when we designed our product,
you know, we are publisher friendly.
We understand that there is no
internet without great publishers.
Like your site is great, it has
a lot of useful information.
Um, and, uh, And so when we worked on the
AI answer, we designed it to be brief.
Um, we want to stay within the
constraints of, uh, of fair use.
Obviously, that's going to be litigated,
uh, in a, uh, in, in a big way.
The reason we put links is not just
so that you can go verify for yourself
that the information is authentic,
um, but we are not saying that this.
Is the end all.
Be all of everything.
If you want deeper insight into
a particular topic, um, you can
follow the citation links, um,
and go to the underlying site.
Um, but I want sugarcoat the fact
that, you know, these kinds of
fluid answers are going to result
in a major, what's the right word?
Re um, you know, Modification
rejiggering, like it needs a new
equilibrium between aggregators
like search engines and publishers.
It is going to be very, uh, destructive.
I think what is going to happen, you
know, we think about this problem a lot.
I think what is going to happen is
that big publishers are going to get
their own chatbots for their content.
The technology that is being used,
uh, to power neva.com can be used
to power search on reddit.com.
In fact, if you, if you, you know, add
Cycle and reddit.com to your Neva search,
we will give you a Reddit summary.
So if you want to know, like, I don't
know, best pressure cookers, but you
want, you know that answer from the
Reddit community, you can just type best
pressure cooker site call on reddit.com.
We are working on just making that plus
Reddit, um, so that you get content from.
So I think there is definitely a
phenomenon by which all of us are going
to expect to be able to talk to any
site to get back fluid responses so that
we are not like, you know, searching
around for what's the information.
I'm sure you've run into cases where
you land on a page, it's not quite what
you want, and then you look at like
the hamburger menu, uh, which, which
page is going to have this information.
It's all very painful.
I think increasingly you're
going to say, you know, Hey, you
better show me that information.
Uh, and if I come to a site and
it's not there on this page, there
should be a great search experience
that will let me easily find
that, that sort of information.
Obviously not everybody is going to be
able to create this technology and create
a big user base that keeps coming back.
Um, so, you know, looking two steps
forward, I do think that there will be
more consolidation of people that are.
Creating, you know, creating
content on our part.
As I said, we try to keep our answers
turfs, get to the heart of what the user
is looking, um, for, and give them ample
opportunity, uh, to go learn more, uh,
from the people that created the content.
Obviously, how Google is going to
do this or how Bing is going to
do this is going to have a pretty
massive impact for what it's worth.
As I said, I expect Facebook,
um, to have a chatbot for content
that's on Facebook similar.
Um, you probably saw, uh, snap
released an AI chat product as well.
I think increasingly we are just going
to expect that we can converse in
natural language with any site, any app,
and get back answers in a format that
we like, that we can easily consume.
It's a big change.
I almost think of this as, as big a
change, say as like, what mobile did, um,
or honestly what even the internet did.
Um, for things like publish.
Kevin Horek: No, I, I a
hundred percent agree with you.
The, the one thing I've been
thinking a lot about, well, I
guess two things is the first one
is getting it personalized to me.
Whether I could ask it questions related
to say like, I'm thinking of buying,
I don't know, a car, for example.
Yeah.
And based on who I am, my lifestyle,
what I like, what I dislike.
Can you recommend.
Which car I should buy within
different price ranges or whatever.
Yeah.
Like is that where we're headed?
Because sometimes people think AI is all
going to doom and gloom and gonna take
over everything and it might wipe out
some type of jobs, but like what's your
prediction or what are your thoughts of
kind of where we, with AI and because
you're actually building it, right?
So many people have these
theories, but they're not
really in the trenches building.
Sridhar Ramaswamy: You're getting
to the heart of where I think
there's going to be change.
Um, all of us have been to sites,
especially government websites,
um, where, uh, they're so finicky
about how you enter information.
I am sure you've run into cases, um,
where uh, if you enter a phone number
without a dash, some sites will get mad
at you and be like, I don't want that.
I hate stuff like that.
That drives me crazy.
Sometimes if you enter the number with the
dash, those sites will be like, nah, nah,
nah, no, you need to have put parenthesis.
Um, it drives you and me crazy.
Um, I think what language models can
do is basically serve as a glue layer
between the information that you
provide and the sites that need that.
This is what they're really
extracting structure is what
they're really, really good at.
It also works the other way.
We are working on, um, on a new
feature to launch as part of Neva ai.
Where we extract things like prices,
availability, um, you know, discounts
different, uh, you know, different
features automatically from webpages
and let you make an easy comparison,
um, to your point about, Hey, can
I just talk to a chat bot and tell
it the kind of requirements that
I have so that it can show me what
cars are most suitable for me?
I don't actually think that
that's, that's far away.
And again, That's the kind of stuff that
these models can be very, very good at.
So for example, I can imagine a
scenario in which you, you know,
you come and say, yeah, I'd like
a four seater kind of a sedan.
Um, you know, and, but you know,
the model might come back and say,
But do you care about mileage?
Do you want, uh, a gasoline car?
Do you want a hybrid or
do you want an electric?
Um, here are like the price ranges for
sedans that in these different types.
Um, and so it is that level of fluidity.
That I think is going to be
really, really useful for us.
And by the way, this is the reason,
um, why the likes of Google and
Bing, uh, should be, you know,
seriously concerned about the future.
Because in a world where there are
answers, where there is a single
answer to a question, um, where
there is an interaction, where you
are giving specifications, And the
expectation is that products are
going to come back or you're going
to be told what's the best for you.
Um, all of the things that made their
marbles very successful, which is.
You know, they can force you to
scan top to bottom on a page.
They can load the top of the page
with like lots and lots of ads.
Uh, and you cannot tell just by
looking at a u r url, whether
that's a great page or not.
All of those things are getting
obliterated by these models because
they can look at the content and
just like, figure out what is
important for you, uh, to see.
That's why I say that this is a time of
just lots of change, not just in search.
In how you'll interact with
email, how you're going to
consume information on websites.
Um, and while we have hooked up a search
engine to large language models, I think
you're going to see these large language
models hooked up to other APIs so that you
can talk and get back stock information.
Um, you, these APIs will
also be able to fetch other
information like, like weather.
Uh, there is a company that is working
on how to drive a browser using
language models, uh, so that if you want
comparisons on two sites, that simply
will not give you the information.
So, for example, in the US uh, the
way you look for a flight is you
can look on Kayak or Google Flights,
uh, for cost information on most.
Except Southwest.
You want Southwest prices.
You have to go to Southwest.
Yeah, but imagine like a language
model that becomes like an
automation layer that says, ah,
Kevin, you're looking for a ticket.
Let me open up two new tags.
I'm going to put your query into these,
get back the results, but I will do the
extraction and summarize it for you.
Right now it's a little slow, but this
kind of tool use, um, is going to be
a big application of language models.
So this is all like, we are very much at
the beginning of what is possible here.
Um, and, uh, you know, yes, there
can always be doom and gloom about
these models hallucinating or doing
weird stuff, but the actually useful
things that they can do, they're just
beginning to scratch the surface.
No,
Kevin Horek: that, that
makes a lot of sense.
It it, it's interesting to bring
the travel example cuz you can
spend, and I do, and we're doing
that right now with booking a trip.
But the, the point is, is if I can
just say, ask it a few questions,
like, okay, we're traveling with other
people, these are the dates I'm free.
They can say, these are the dates, they're
free, and we're saying these are the
destinations we want to go, and then it
starts spitting back information to me.
Maybe it's live, maybe it's like we need
to compile this over days because we
need other people to, to give answers to.
But how does that, I guess,
play into the privacy side of
kind of Neva and all this stuff?
Because obviously if I'm giving the data,
then obviously I'm fine sharing that.
I'm the type of person that certain
companies, I will give as much data as
I can because I want that convenience
that they're gonna give back to me.
And then there's other companies where
I'm like, I don't wanna give you anything
because I don't like that company.
And so how do you manage that in kind of
ai, Neva and kind of the privacy side of.
Sridhar Ramaswamy: Uh, so our privacy,
uh, rules are still the same, which
is we don't collect, uh, search
history unless you go turn it on.
Um, we do not use your information,
obviously, to show ads or affiliate links.
Uh, and, uh, we don't sell information.
We want, uh, you know, uh, like
people that get value from Neva,
we want you to pay for the product.
Uh, and rest easy.
That, that is like, that
is the relationship.
It's a very simple, um, relationship.
I, I have told people that my aspiration
is for Neva to be like, tap water, uh,
high quality, low cost, always just there.
It works, it's predictable.
Um, and.
So there are privacy concerns with
large language models, and at NEMA we
are very careful about how we use them.
Uh, so for example, um, you don't want
your private data, uh, to be fed into,
uh, a shared model and used for learning.
Um, that's your private, um, you
know, that's your private information.
Uh, and.
So when we train models, for
example, we trend train them only
on publicly available, um, data.
And so we haven't, we are working on a
feature, for example, to like summarize
a Google Doc that you will have.
But when we do the summarization,
um, those, you know, The content of
that doc is never put into the model.
It's never put into, into a way that can
invade your, that can invade your privacy.
Uh, so I think there is, uh, you know,
there's more stuff that is going to
go on here, uh, even from like an
enterprise viewpoint, for example.
People are not going to be very happy if
their proprietary documents are fed into
like a publicly used, uh, um, used model.
So I think there's going to be a lot
of innovation that has to happen.
The way we think about it is,
um, base models, uh, can only be
trained on publicly available data.
Um, and then we use a particular
technique, um, it's called, the technical
term is retrieval augmented generation.
Essentially, we use the context of
some results in order to construct an
answer, um, for you and, uh, um, that
we do it carefully so that it does not
leave, um, any imprints on the model.
Um, the, uh, so for example, if you.
Even now, the way it works is if you
type in Kevin Harrick into niva, we
will use the content of your site
in order to generate the answer.
Um, but that content is not really
going to be logged anywhere.
So there are privacy
Safeway of doing this.
Um, but you know, a lot of people are
concerned and we should continue to pay
attention to how our data is being used.
Kevin Horek: Interesting.
It's also such a departure from kind of
how we've traditionally used the internet.
Like I.
We could argue about Apple's choices
around privacy, but I think they
were kind of one of the first big
companies to really push for it.
And so I think the fact that
they pushed for it, I think a lot
more people are really thinking
about their privacy online.
Do you agree with that or what
are your thoughts around that?
Sridhar Ramaswamy: Uh, actually I
think it's kind of sad that a far
profit company that makes a lot of
money with ads is the one that is the
biggest sort of advocate for privacy.
For you and me, that's
the government's job.
Uh, but still I agree with
you, um, that they have pushed
privacy forward, uh, a lot.
Uh, and, uh, um, I think like this
more and more needs to become the
norm for how products operate.
Uh, you know, by.
All of your listeners have, uh, you
know, heard this phrase, uh, if you
aren't paying, you are the product
that really, really, um, is, is true.
There's no free lunch in, in this world.
Um, and so it is something to
keep in mind, uh, as we figure
out like where to spend our time
and what kind of products to use.
No, that makes
Kevin Horek: a lot of sense.
So how do you bridge the gap though?
Because the internet basically started
out as free and a lot of people.
You know, they'll go, and I'm guilty
of this sometimes too, is, you know,
you'll go buy like an $8 cup of coffee
or specialty coffee or whatever, but
spending like 99 cents on an app that
you use all day every day and you know,
is like people frown upon that, right?
Like how do you bridge that gap between
making you not the product anymore
and actually paying for that things?
Sridhar Ramaswamy: I think
there'll be a slow transition.
I think companies like
Apple are going to lead the.
Uh, as you know, whether you like
it or not, you're likely paying for
storage, uh, on your, on your iPhone.
Uh, I think they will start
adding more and more products.
The nice thing about this moment
and large language models is
that they're so expensive.
General, in general, purpose models
are really, really expensive to run.
Um, that is not really free, uh, not
really feasible to have them be free.
I think it offers, it
offers the opportunity for
creating bundles of things.
Uh, with Neva itself, for
example, when you pay for Neva,
you also, um, get, uh, a V P N.
You get, uh, you get a password
manager for a limited time, but you
get these things in, in addition.
Um, I think, uh, you know, just like we
subscribe to a cable package, I think
for a set of essential services on the
internet, there'll be an internet package.
This sort of change takes, you
know, takes a while to come, but
I think it's very much there.
Yeah.
No, I hundred percent
Kevin Horek: agree with you.
So I'm curious, I want to dive a little
bit deeper into any other thoughts around
AI and does machine learning play into ai?
Because they're both kind of
buzzwords right now, and I think
people don't really know if they're
the same, if they're different.
What are your thoughts around those
and how are they similar or different
in what you're trying to do with ai?
Sridhar Ramaswamy: Well, uh,
you know, there is a, there is
a gray line between the two.
I would say the, uh, the defining moment
for large language models, um, for
machine learning in general, or deep
learning as it's called, using these
constructs known as neural networks.
Um, I think the semial.
Where, uh, people were like,
oh my God, this is incredible.
Uh, happened a few years ago, um,
when, uh, it's actually open ai.
Uh, when people at Open AI realized
that G P D three, which was the third
generation of this model that they had
trained, could solve dozens of problem.
That used to be specialties.
There used to be groups of people that
specialized in things, like one group
would be working on question answering.
Another one would be working
on sentiment detection.
Is this text angry?
Is it sad?
Is it happy?
And then some other people would
be working on machine translation.
How do you translate from like
English to German or something else?
These people realized that, um,
at some point the models got large
enough and smart enough that they
could solve dozens of problems that
previously need specialized people and
models and training for in one shot.
In my mind.
Um, that's the aha moment.
Um, and so again, the technical term,
um, is, uh, you know, the, the title of
their paper is something like GP Open.
AI says G PT three is a general purpose,
few shot learner, meaning that you can
give it a couple of examples and it'll
instantly know how to solve that problem.
If you, if you tell G P D three, you know,
here is how you translate from English to.
Or English to some other language.
Um, and, uh, oh, translate
this piece of text for me.
It's able to just go ahead and do that.
Um, that was a real breakthrough moment.
And you can say like, in some ways,
um, that is the beginning of the
revolution that is just getting started.
Um, so as I said, there's a bloody line.
Um, but, uh, what people are realizing
is that they can build models with these
powerful properties, uh, first of all
in language, which is going to have a
big profound impact because language
embodies so much of human's, wisdom,
humanities, communication, and so on.
But there are also, uh, models
now that, uh, are able to do
things like image generat.
And video generation models
are also quite close.
Uh, and then there are other things
that, you know, can take text, uh,
and image and generate related images.
Um, so there's a lot that is going
on in the world of, uh, of ai.
Um, but I would say like being
able to solve a number of.
Like dozens upon dozens of language
problems, um, was the breakthrough
moment where everybody went, wow, that
is indeed like artificial intelligence.
But it turns out intelligence
itself is complicated.
And so OpenAI and many of these
other labs are working on what they
call agi, uh, which is general,
uh, you know, intelligence.
Uh, it's the kind of stuff that you and
I do, um, which is being able to think,
being able to reason, being able to,
um, learn, uh, you know, new things.
Uh, so this area is very rich.
There's a lot more to go.
So how
Kevin Horek: do you guys stay
on top of this being one of
the first to market really?
Sridhar Ramaswamy: It's, uh, uh, you
know, there's a lot that is going on
with, uh, um, with large language models.
And so we have a study group.
Uh, we meet, uh, every week or every
other week and we discuss new papers.
We are not a research lab, so we do have
to be careful about what we work on.
So we always have an eye on, um, what
can we apply, what are the biggest
problems that, um, you know, that we have.
Uh, and um, um, and so it is a
continuous iteration process.
Um, as I said, we are currently working,
uh, on language models that can do
things like understand product pages,
uh, and extract information from them.
Um, this is after some of the
text problems are, uh, are, you
know, are are taken care of.
We are well on our way.
Um, it is, it is, it is
hard for a startup to do it.
Um, but on the other hand it is, uh,
we are not a, we are not open ai.
Um, we are not a general intelligence
agi kind of, uh, company.
Uh, so we often will run smaller
models than the biggest ones that
open AI or philanthropic will run.
Uh, they save us money.
Um, they are also completely in
our control because we run them
as part of, uh, um, our systems.
So we keep a sharp lookout for
great ideas that are coming and
stay like half a generation behind.
Uh, so that we get all of the benefits
of new things that come out without
quite like the cost factor that
would be very prohibitive for a.
No,
Kevin Horek: that makes a lot of sense.
So how is Neva AI similar and or
different from chat G P T and what
Microsoft is doing and, and what Google
is rumored to be releasing any day?
Any time now?
Yeah,
Sridhar Ramaswamy: yeah, totally, totally.
Um, as I said, chat, g p T is
what I call open loop generation.
Uh, so the way you talk to it, you
ask a question, it'll generate an.
These answers, uh, will
always look pretty good.
Uh, but you don't know, um,
whether they are real, uh, or uh,
or are false, and they make no
claim to it to be fair to them.
Uh, chat GD is amazing
for creative use cases.
Um, I don't know if you have tried
writing poem, so charge G P T, um, I.
And I've sort of like made it almost
like this interactive process where
I'll be like, well, I wanna write a
poem about, uh, Kevin, um, use these
other words, um, uh, you know, that
rhyme with his name, uh, and, uh,
make it a, you know, make it a limit.
And so there's like something
that it'll generate.
You can, you can tinker with it
and say, I don't like that line.
Let me change it to this line.
And so for those kinds of use cases,
it is truly, truly magical, um, to
interact with a model like this.
I, again, you can also write
fun short stories, um, with it.
When we designed Neva ai, we said we love
the fluence fluency of chat G p T, but we
want our AI to be referenceable, uh, so
that people can know where we got it from.
We wanna make sure that
it is high quality.
We don't want to be like making up things.
We also want it to be, uh, real.
Uh, and uh, so we came up with a
way, essentially to run a search
engine and these large language
models in parallel at the same time.
And that's the product that you see.
Um, obviously, uh, the big
companies, Google and Bing.
Also want to launch this.
Um, Bing has a system that is, uh, that
is, uh, quite good for, uh, for search.
It's very slow.
It takes like 7, 8, 9
seconds to give an answer.
Um, we basically said it's not
okay to spend more than 500
milliseconds, um, extra in order
to dr in order to generate ai.
So we had to resort to little hacks,
like stream the answer out to you.
Uh, we are working on, uh, doing all of
the work completely in-house so that we
can generate the final answer as well.
Um, within like, you know,
500, another 500 milliseconds.
Uh, so we think we have, uh, uh, a fair
amount of headway in what we can do.
We are also looking forward with
glee, uh, to commercial area.
Where we are free to run aok and create
incredibly useful products that Google
and Bing are never going to touch because
they would lose money handover fist.
So there's a lot of white space in
terms of where can we create value
for you, um, our Lyle user in ways
that the commercial search engines
are not really going to do quickly.
Kevin Horek: Can you maybe
give us some examples of
Sridhar Ramaswamy: that?
Uh, so for example, if
you look for a product.
Um, we can tell you, oh, uh, you
know, here's information about this
camera that you're looking for.
I'm happy to send you screenshots.
You can put them in
your, um, episode notes.
Um, sure.
Yeah.
So actually it's, uh, you, you
edit this, so let me share.
Uh, something.
So for example, uh, you know,
here's, here's one case.
Um, uh, this is a mock.
We are working on it, so if you look
for this camera, we will generate
text, but we'll also extract out all
the important features that you want.
We'll, also, Compare across pages
and be like, ah, this is where
you can buy the product from.
We will extract pros and cons so that it
is an incredibly quick summary of what
you wanted to know about this camera.
On the other hand, if you ask a
broad query, um, we'll be like, okay,
these are the top four headphones.
These are the pros and
cons of each of these.
Um, and yes, we can also show you
where to buy them, but we can generate
experiences like this using the same
technology we think for queries like this.
Neither Google Naing is going to be
anxious to produce these kinds of
answers that have no ads in them.
Here is another example back
to your idea of an assistant.
Um, this will probably
be more interactive.
You type in something like auto insurance.
Um, we'll be like, well, there are
different kinds of auto insurance.
Which one are you interested in?
Uh, and then we'll go back and say, uh,
if you're wondering about how much your
auto insurance will cost, here are the
factors that are going to influence.
And here is now a table of the
different people that can give
you insurance in your area.
Um, and these are the ratings from users.
Um, and so you can, like, this is
genuinely a commercial assistant.
These are the kinds of cases, as
I said, where an ad-free search
engine can just like go crazy
innovating in these areas because the
technology is really that powerful.
Kevin Horek: No, that's
actually really cool.
Like that's basically
what I think everybody.
Or wanted Google Assistant
to be or Siri to be, right?
That's correct.
But it's nowhere near that
Sridhar Ramaswamy: because they
didn't have the technology.
Um, you'll find it funny, but, uh,
early versions of Google Assistant,
um, until very, very recently.
Um, They did not, their, uh,
language understanding was not
sophisticated enough that they
could answer, follow on questions.
Um, well, so for example, um, if
you ask any of these assistants,
um, who's Barack Obama?
They'll tell you, blah, blah, you
know, president of the United States.
And then if you follow it up
with, okay, who are his children?
Um, basically the way Google solved
this problem six years ago was by
looking at logs and saying, oh, people.
Um, that type for Barack Obama and
then type who are his children, um,
you know, and then spoke who are his
children will correct themselves and
say, who are Barack Obama's children?
So it would like learn these associations.
On the other hand, you can try this in
chat, G p T, um, where, uh, uh, if you
put in one query and ask a follow-up
query, it uses the context of the previous
query to determine the current one.
This sort of technology didn't really
exist seven, eight years ago, and that is
part of what is exciting because it is a
conversation with memory, with context,
but it can be turned into really useful
products that you and I are going to love.
Kevin Horek: No, totally.
Well, and it saves a ton of time, right?
Because, uh, well I, maybe not everybody
does it, but I do that all the time.
Like in your screenshots you just showed,
it's like if I'm buying headphones, I'm
comparing four or five versions, right?
And if I can just do that
in search results, you know,
you're gonna save users.
A ton
Sridhar Ramaswamy: of time,
ton of time, ton of money.
Um, and, uh, and, and
it's also a lot of agency.
You know, you get to do what you
want, uh, to do and, uh, is like the
things that you see are not being
dominated by, you know, I don't
know, silly ads with geckos on them.
Remember, how do you buy insurance?
Uh, we listen to people
who sing, uh mm-hmm.
We listen to funny lizards that do weird
things on the screen or to basketball.
Uh, you know, stars talking about how
they need to give each other an assist.
That's how we buy insurance.
Like, uh, uh, okay.
Kevin Horek: Yeah, it's
interesting, right?
Because you never Yeah.
Because if you're not, you're giving me
honest results about what makes sense
to me based on what I'm searching for.
Yeah.
That's, this is like game changing
Sridhar Ramaswamy: really.
That is again, the, um, the
business model, the technology
is all getting there.
Um, it's a matter of like putting
it together fast enough that it
delivers like a ton of use for people.
Kevin Horek: No, that
makes a lot of sense.
And paying for that a few dollars
a month is a no-brainer, right?
That's right.
Especially
Sridhar Ramaswamy: for a, that's
when, that's when the utility really,
really gets, uh, you know, get.
Sure.
Kevin Horek: So I wanna just, for
people that maybe haven't heard of
Neva, I want to cover some of the
features, uh, like the Chrome extension.
Yeah.
Uh, some of the other features that
you offer, and then the apps, because
I like, there's more to it than just
like replicating Google, deleting
the ads and, and making it private.
Right.
So do you want to dive a little bit
deeper into that and then maybe how AI.
Encompasses
Sridhar Ramaswamy: all
of the supercharges.
Yeah.
Yeah.
Uh, so, you know, when we said about to
create Neva, um, we really wanted to, um,
like make, um, your interaction with the
internet more straightforward, more fun.
I did score.
Neva is a search engine.
You type in a query you
get, you should get.
Great results.
That's like the baseline aspiration.
So sorry
Kevin Horek: to interrupt you there.
Yeah, please.
I, I think it's important that
because I, one of my favorite features
of Neva is you, I had to add it
myself just for a caveat to this.
Yeah.
But it also searches like my Google
stuff, my Dropbox, my Figma files, like
a bunch of third parties that I had.
Request, like give permission to Yep.
And I love that feature that I have
not seen anywhere else, but continue.
Sridhar Ramaswamy: Yeah, yeah, yeah.
Uh, so we start with like,
Hey, great public search.
Um, and then we looked at, uh, how
can we make this even more useful?
So we added features for personalization.
You can say things like, I prefer reading
this newspaper because that's the one that
I'm actually paying a subscription to.
Hyper for the New York Times
and the Wall Street Journal.
All things be being equal.
I wanna see more of them.
You can also do things when
you look for health queries.
For example, you can say, I only
want to look at information.
From trusted nonprofit and government
websites, um, and not from, you know,
like lifestyle, uh, websites that will
try and sell you all kinds of snake cards.
So you are very much in control
of the search, um, the core
public search experience.
As you were saying, Kevin, you can
also optionally do other things like
connect your personal accounts, whether
it's Google Drive, office 365, um, or
Dropbox, and be able to search all.
I don't know about you, but
my life runs on Google Drive.
Like all my tax stuff, my wrist receipts,
et cetera, they're sitting on drive.
My pictures are there all the, you
know, documents from my life are there.
Um, and so if I am, uh, you know,
getting ready for a trip and want to
enter my passport number somewhere, I
just would type like passport number.
Inva and I'll be pointed to
the P D F of my passport.
I also have a spreadsheet
in which I keep this number.
All of this stuff, it is truly search
or the things that matter to you.
Um, and so the way people use Neva
is either by installing a Chrome
extension, Or by using our apps on iOS
and on uh, and, and on Android and in
all of the products we make, we ship a
technology called tracking prevention,
where we basically prevent third party
sites from snooping on your behavior.
So if you go to cnn.com, uh,
CNN knows what you browse there,
obviously, because it's their
content, but hundreds of other people.
Put little pieces of code on CNN
so they can keep track of what
you're doing and come up with a
profile of all of your activities.
Across all sites.
We wanted know part of that.
And so part of the technology that
ships in our extension on Chrome, for
example, um, is tracking prevention.
We stop people from being able to
track what you do across sites.
This means that those annoying
ads that keep chasing you around,
they are a thing of the past.
Um, so those are sort of some
of the key features of Niva.
And what we have done now with
AI is put in the final layer.
We take all these first results and
produce answers for you so that at a
glance you get like the first answer
to a question that you have put in.
We have not yet launched answers on
personal data that's only like a month
or so away so that, um, in our ideal
world, You know, if I were to look for
Kevin Huk, um, in my Niva account, you
should be able to say, Hey, these are
the four meetings that you have had
with Kevin over the past four years.
These are the email exchanges.
Um, again, all done in a private Safeway.
We think we are well on her way to truly
creating a personal assistant for you.
Um, that is pretty fanatical
about protecting your
privacy, protecting your data.
And having a really, really
simple business model.
No,
Kevin Horek: I, I hundred percent agree.
Like I almost use it as
like my dashboard, right?
Like just neva.com and just because
it gives you, the other thing too
is you pulls in, you mentioned news,
but you can get like weather and kind
of like package deliveries are nice.
Like you have a bunch of stuff
built in, uh, that it's more like
a dashboard that I can like search.
Is that a good way of explaining?
Sridhar Ramaswamy: The homepage 100%.
Um, that's sort of, you know, that is how
we, uh, designed it, but a lot of our use
cases come from people simply putting in
that query, um, and getting to all of the
information that matters in their life.
Yeah, that,
Kevin Horek: that makes a lot of sense.
So I'm curious with kind of the AI
side to kind of come back to that.
How do you, I
Sridhar Ramaswamy: guess,
Kevin Horek: keep it going, I
guess, because there's so much
infinite possibilities with
this, and how do you decide?
Where to take the roadmap because, and,
and I message you about this, the second
I think you emailed about that you
were launching Neva AI is like, can I
integrate Neva AI into my third party app?
Right?
Like, there's so many different
ways that this can go.
Like how do you manage that
roadmap and like, will you let
people like myself integrate it
into my own app down the road?
Or, or where do you see
the future of, of this go?
Sridhar Ramaswamy: Part of what we've
been doing is we've been building
out this tech, um, ourselves.
Uh, our overall search system is in
very good, uh, is in very good shape.
Um, it, uh, has excellent crawl
coverage, excellent quality in serving.
Uh, we are, uh, uh, you know, have also
been developing, uh, AI technology,
large language models that we train,
that we run in our own clusters.
Uh, and there is a real hunger.
For programmatic access, API
access, uh, to this functionality,
we are absolutely working on it.
We are also in the process of embed.
Um, our AI technology into this
product called PO P o e, uh,
that Qura released recently.
It's a, it's like a chat bot aggregator.
You can talk to different chat botts
from inside, uh, from inside po.
Uh, so definitely making the
technology broadly applicable.
It's something that we are very keen on.
And I also told you, um, you
can search over Reddit from Neva
simply by, you know, appending
site call and reddit.com to that.
You can also do, you know, search
over New York Times by appending
site call and ny times.com.
And so we realize that there is now this
opportunity to provide a conversational.
Chat like experience to pretty
much any site on the planet
because we are already doing it.
And so how we license this
technology is an active topic.
We are busy creating APIs so that
people can, uh, um, can use, uh, both
our search and our AI functionality.
Um, it's, it's an exciting area and,
uh, yeah, we are a 50, you know, we are
a 50% team, and so just prioritizing is
the biggest issue that Wewe and I face.
Yeah.
Kevin Horek: That makes a lot
of sense, but we're kind of
coming to the end of the show.
I I, is there any advice that you would
give to companies or entrepreneurs that
are in the AI space that you've maybe
learned that you'd like to pass along?
Sridhar Ramaswamy: Um, it's very, uh, you
know, uh, uh, like attempting to think
that AI is going to solve all problems,
uh, AI is an incredibly powerful.
But you still need to sweat the
details of how are you creating value,
how do you identify a need, um, how
do you go about creating a product
that is going to have, um, you know,
people actually adopt it, uh, and then
turn around and uh, and pay for it.
And also if you are a really, really thin
layer on top of open, uh, you know, open
ai, um, there's not much that stops other
people from creating clone products.
Um, but what is hard to do even
today, um, is creating customer value.
So I would say the, the basic
qualities of it is really,
really important to understand.
Your customers understand their
pain points, understand where they
are going to create value and make
sure that your product works tightly
with that, um, is very basic advice.
But I would say even in this
AI world, um, that is, um, that
is something to keep in mind.
The nice thing is that it
is wonderful to get going.
Um, with open AI's APIs, you
can do that very, very quickly.
Go build p.
I think that is actually,
uh, that's a lot of fun.
Um, but I would, I, I would focus on,
um, what are we doing with customers?
Where can we create value?
No,
Kevin Horek: I think that's really
good advice because when there's a
hot new technology, I've even heard
it from people that I've worked
with, it's like, how can we integrate
chat g p t into what we're building?
It's like, Do we need
to even integrate it?
Like
Sridhar Ramaswamy: it
doesn't add any value.
Create more value.
Yeah.
How can you create value for your
customers and what opportunities,
um, do large language models, um,
and whether it's in-house or whether
it's chat, g p t, doesn't matter.
Um, like where can they be
used to create even more value?
In my mind, that's the biggest
question, um, for people to continue to.
Yes, knowing these models, knowing
the APIs, being able to quickly
put things together, those are
wonderful, those are wonderful skills.
Um, but, uh, you still need to
solve the basic problem of like,
what do your customers want?
Where can you create value for them?
No, I think
Kevin Horek: that's really good
advice, but sadly, we're out of time.
So how about we close with mentioning
where people can get more information
about yourself, Neva, and any other
Sridhar Ramaswamy:
links you wanna mention?
Uh, absolutely.
Uh, uh, please go to neva.com.
Uh, you can, uh, you know, uh, you can set
up an account and get going right away.
It's a, uh, it's a premium model, so
it's a free service, uh uh, or you can
download our app either on the Play store.
Or on the App store, on, uh, on, on iOS.
Uh, we are just getting started.
Um, you know, your listeners are
going to be amazed, um, by how we
have sort of brought forth ai, um,
in, uh, in, uh, uh, an authentic
but really, really easy to use way.
And as I said, you're only getting
started, the product is getting
better, um, by the day, niva.com.
No, I, I,
Kevin Horek: and I, like I said earlier
in the show, I've been using it.
Pretty like a year or so and how much
features you've put in over the last
year is actually pretty incredible.
Like, I was thinking about
that before I was getting on.
I was like, that wasn't
there like a year ago.
Like, you know, just how much it's grown
and is, is been really cool to watch and
Sridhar Ramaswamy: see.
Right.
So it's, it's uh, it's a good
moment to be a technologist.
It's a good moment when there's
like, you know, a tsunami shows, uh,
shows up and you're able to surf it.
Um, sure.
So these are exciting times for all of us.
Kevin Horek: Very cool.
Wellar, again, I really appreciate
you taking the time outta your day
to be on the show, and I look forward
to keeping in touch with you and
have a good rest of your day, man.
Sridhar Ramaswamy: Thank you, Kevin.
Take care.
Thank you.
Okay.
Bye.
Bye.
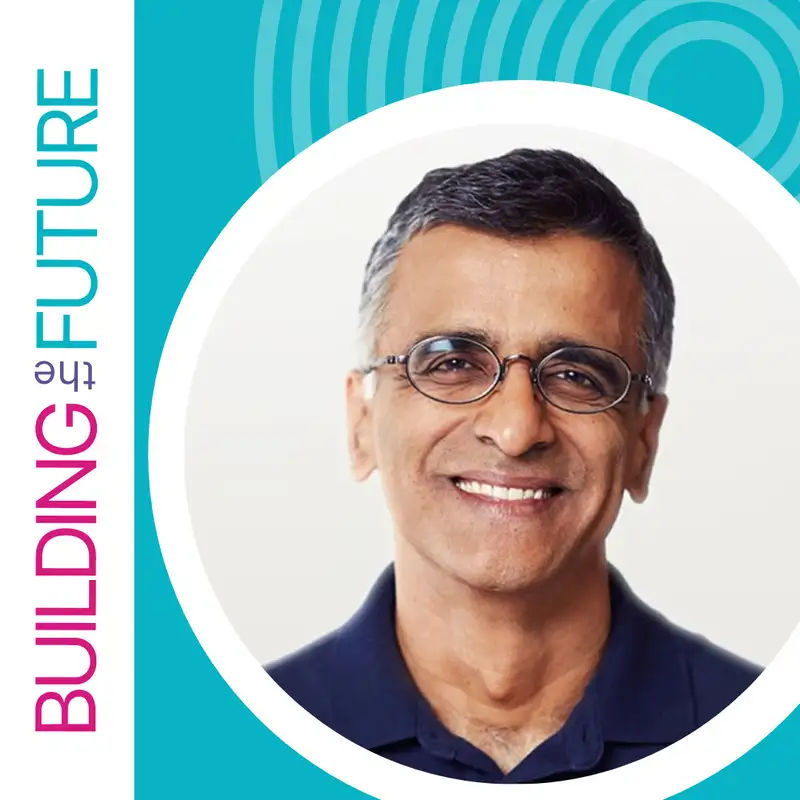